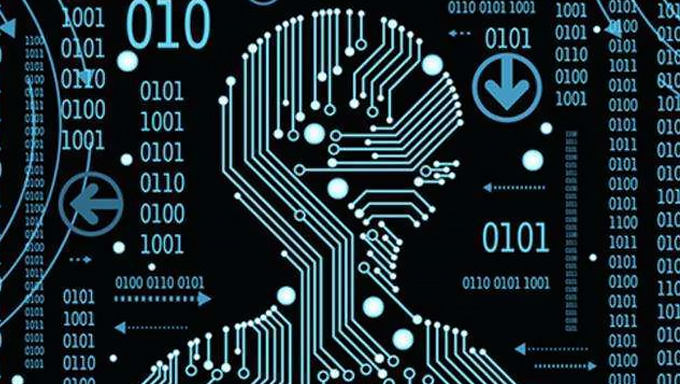
-------------------课程目录-------------------( U) A' Y4 b5 G8 Q
2 A0 M3 I5 {# B$ F( U$ Z
01_handout.pdf7 q& A7 g9 I6 u- ]5 Y( }
02_handout.pdf6 H5 {. k5 ? C: Y! [- u) @: _
03_handout.pdf
04_handout.pdf, A+ j. } P. o+ d2 |
05_handout.pdf
06_handout.pdf
07_handout.pdf
08_handout.pdf4 l% U$ a" ~! u) R5 W4 P; s* T" i
09_handout.pdf/ M5 B& O0 m6 B! N. N
1 - 1 - Course Introduction (10-58).mp4
1 - 2 - What is Machine Learning (18-28).mp4& J! d7 K" E+ J. k7 B
1 - 3 - Applications of Machine Learning (18-56).mp4
1 - 4 - Components of Machine Learning (11-45).mp4
1 - 5 - Machine Learning and Other Fields (10-21).mp40 o$ l: s9 D: B3 n
10 - 1 - Logistic Regression Problem (14-33).mp4
10 - 2 - Logistic Regression Error (15-58).mp4
10 - 3 - Gradient of Logistic Regression Error (15-38).mp4
10 - 4 - Gradient Descent (19-18).mp44 u* J" P* Q$ j' `
10_handout.pdf8 i& V. f: s( b0 z# Q6 U
11 - 1 - Linear Models for Binary Classification (21-35).mp4
11 - 2 - Stochastic Gradient Descent (11-39).mp4
11 - 3 - Multiclass via Logistic Regression (14-18).mp4: \9 X1 v! h5 q4 ]5 t6 c8 J, v
11 - 4 - Multiclass via Binary Classification (11-35).mp4
11_handout.pdf0 L* x5 E- i, _% V1 Q
12 - 1 - Quadratic Hypothesis (23-47).mp4+ u B, X8 Y- C4 ?9 j7 n$ v) Q- j9 B
12 - 2 - Nonlinear Transform (09-52).mp4
12 - 3 - Price of Nonlinear Transform (15-37).mp4
12 - 4 - Structured Hypothesis Sets (09-36).mp4
12_handout.pdf
2 - 1 - Perceptron Hypothesis Set (15-42).mp4
2 - 2 - Perceptron Learning Algorithm (PLA) (19-46).mp4
2 - 3 - Guarantee of PLA (12-37).mp41 a) Y* |/ G5 m& m
2 - 4 - Non-Separable Data (12-55).mp4/ f) } y/ {& c6 n2 j! m
3 - 1 - Learning with Different Output Space (17-26).mp4$ Y4 ?; q, {! t" T4 B+ U
3 - 2 - Learning with Different Data Label (18-12).mp4: m6 c2 r! o; Y/ G6 B
3 - 3 - Learning with Different Protocol (11-09).mp4
3 - 4 - Learning with Different Input Space (14-13).mp4
4 - 1 - Learning is Impossible- (13-32).mp4$ b8 y# b" E; k3 m
4 - 2 - Probability to the Rescue (11-33).mp4
4 - 3 - Connection to Learning (16-46).mp45 j. v. R4 R7 U% @6 ?
4 - 4 - Connection to Real Learning (18-06).mp4& I" `( m4 s* F9 P
5 - 1 - Recap and Preview (13-44).mp4$ t/ }! m7 y6 M% o
5 - 2 - Effective Number of Lines (15-26).mp4
5 - 3 - Effective Number of Hypotheses (16-17).mp4
5 - 4 - Break Point (07-44).mp4
6 - 1 - Restriction of Break Point (14-18).mp4
6 - 2 - Bounding Function- Basic Cases (06-56).mp4
6 - 3 - Bounding Function- Inductive Cases (14-47).mp4
6 - 4 - A Pictorial Proof (16-01).mp4- F; g8 m5 i+ S2 D: H
7 - 1 - Definition of VC Dimension (13-10).mp4
7 - 2 - VC Dimension of Perceptrons (13-27).mp4
7 - 3 - Physical Intuition of VC Dimension (6-11).mp4 M5 R+ E2 B4 x8 V" B5 E9 ]
7 - 4 - Interpreting VC Dimension (17-13).mp4* e. a% x+ l# y* R/ w7 h& P- V
8 - 1 - Noise and Probabilistic Target (17-01).mp4
8 - 2 - Error Measure (15-10).mp4$ F: | |' @! O, u9 g: E7 d1 f
8 - 3 - Algorithmic Error Measure (13-46).mp4- e# E" Y5 [5 Z& p+ w8 T
8 - 4 - Weighted Classification (16-54).mp40 f0 r* ~3 O, r. ]% J% ~7 `- |
9 - 1 - Linear Regression Problem (10-08).mp4
9 - 2 - Linear Regression Algorithm (20-03).mp4
9 - 3 - Generalization Issue (20-34).mp4+ c) V9 V. A! A. f' N
9 - 4 - Linear Regression for Binary Classification (11-23).mp4
HomeWork1.doc
homework2.docx
homework3.docx
相关资源